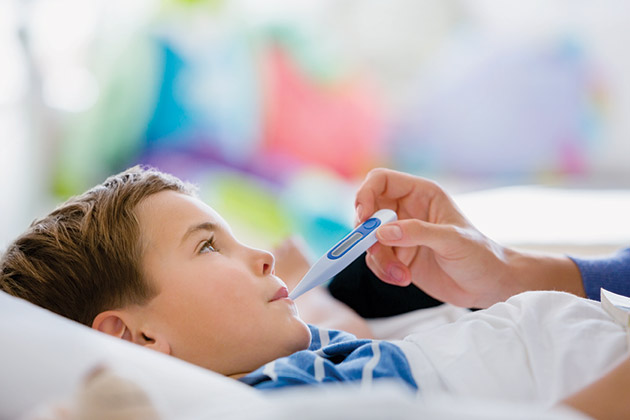
Despite rapid advances in technology, pharmacovigilance (PV) units still handle the majority of adverse event and safety information data collection and processing through manual efforts. Once the data is captured, it is often stored in siloed databases making it difficult if not impossible to aggregate and analyze in meaningful ways.
According to the European Medicines Agency (EMA) and the US Food and Drug Administration (FDA), the amount of adverse event reporting is increasing by more than 15 percent annually. Adding to this complexity, the number and variety of data sources PV units are expected to review is growing exponentially, making it increasingly difficult for PV professionals to keep up.
Many of these data sources are unstructured in nature where information has to be culled from narratives rather than directly lifted from forms and reports. These unstructured sources include emails, social media posts, journal articles, audio files, and handwritten documents, some of which require language translation prior to extracting relevant details.
The deluge of new data, increasing requirements from regulators, and declining PV budgets are forcing the PV community to seek new solutions to improve the access, timeliness and cost-effectiveness of safety data management.
THE ARTIFICIAL INTELLIGENCE EVOLUTION
Advances in automation, machine learning (ML), and artificial intelligence (AI) can address the challenges in PV while enabling new levels of insight and proactive analytics to enhance quality and oversight for PV leadership. Many industries have already proven that AI and automation can deliver improved speed and quality in a variety of applications – the same benefits can be achieved for drug safety.
To ensure success, it is recommended that teams begin with small targeted automation projects and progress over time to larger and more complex initiatives. In affect taking a crawl, walk, run approach to automation adoption.
CRAWL
In the crawl phase, it is recommended that teams identify high volume, labor-intensive, and repetitive tasks that, if automated, could have a meaningful impact on a team’s overall productivity. These early wins can inspire the staff to embrace additional opportunities that may be more complex to automate.
For example, many organizations begin with case processing activities, including PV intake, as this is where the majority of today’s PV staff are engaged. Using automation tools, adverse event information can be captured directly from structured web forms, apps, XML documents and other structured formats and automatically uploaded into safety databases. This drastically reduces, if not totally eliminates, the need for human effort in these tasks. This simple application of AI in PV can deliver significant time and cost savings, while reducing the risk of error associated with intake tasks.
Early wins at this stage not only reduce processing time but eliminate steps, which very much reduces cycle time. In the current extreme workflow-driven systems, a case runs through at least five workflow steps and is handled by multiple associates with different skill sets, raising the risk of human error. The total time spent on these tasks averages around 150 minutes over the course of five business days before a case is fully processed. Eliminating an entire step reduces that risk and cycle time substantially.
WALK
Natural language processing (NLP) and optical character recognition (OCR) tools can deliver further productivity gains for PV tasks by translating unstructured data into structured formats ready for processing. Natural language processing tools can read source documents and identify relevant information within unstructured content, which can then be transformed into key data elements and aid in the creation of clinically robust auto narratives detailing the clinical story. This eliminates the need for labor-intensive and time-consuming data entry and authoring. Through the use of machine learning, machines can be trained
to identify data patterns and how data elements should be handled based upon previous examples. In effect, the machine learns what to look for and how to process the data correctly in future cases, making it smarter over time. As an example, the use of auto-narrative tooling can lead pharma companies to realize significant reductions in labor for case-narrative creation. Our experience demonstrated that manual intervention is not needed in up to 75 percent of auto-narrative enabled cases.
RUN
Artificial Intelligence enables the machine to perform cognitive tasks and validate decision-making on its own. This enables progression towards zero-touch case processing goals for our industry. Advanced capabilities, such as predictive data analysis and signal prediction, fall into this category. For example, advanced cognitive tools can perform data listening to monitor conversations on social media and other platforms around the clock, without human intervention, ensuring adverse events are promptly identified.
Another area where AI tools are being deployed is in auto-translation. Source documents can now be automatically translated bi-directionally from source language to English or vice versa, presenting a machine- translated document at high-speed and accuracy. Machine translation removes human variability and reduces overall translation timelines. NLP can be applied to the text to extract relevant safety details for case processing and global PV reporting. At IQVIA, early analysis of our AI translation tool was found to have 85 percent initial machine accuracy for certain language capabilities. Our neural machine translation (NMT) solution maintains the document formatting, delivering a translated document with the same rendering as the submitted file. Continuous machine learning allows translated documents to incorporate preferred in- country speech patterns. Twenty-five languages are planned for IQVIA’s NMT solution by the end of 2019.
SMART ENOUGH TO FAIL
All of these automation tools have the potential to make decisions about safety data independently. However, it is critical that both the machines and humans recognize when confusing or conflicting data requires human intervention. This machine/human interaction is a vital component to the success of automation adoption as it allows companies to properly address potential risks and clearly outline when human intervention will be required to make complex decisions and properly complete required tasks.
CONCLUSION
LAYER UP
As the above examples demonstrate, automation isn’t a single application that can be applied to solve all one’s automation needs but rather a combination of multiple tools and technologies leveraged together within the PV ecosystem to automate safety tasks, reduce risks, and make required safety data more easily available. The most effective systems will leverage a rich combination of these tools within a single platform that can review and digest large global data sets, and use predictive analytics to determine outcomes. When these steps are taken, PV professionals can draw the appropriate conclusions and drive more sound decisions regarding patient safety.
Automating pharmacovigilance isn’t an inexpensive or quick journey, but the end results will deliver a better, faster, more robust and sustainable system for all, including patients, and in the end isn’t that what PV is all about?